Post by Admin on Mar 12, 2021 4:29:42 GMT
DNA Methylation and Phenotypic Inference
Post mortem DNA decay, especially cytosine deamination, is
known to have a differential impact on methylated and unmethylated
CpGs.46 This provides an opportunity to map DNA methylation
marks on ancient genomes, assuming USER treatment of
DNA extracts47 and sufficient sequencing efforts.48 We leveraged
the two high-coverage genomes characterized here and
four others previously reported4,12 to identify genes that may
have experienced important DNA methylation changes during
the Mesolithic to Neolithic transition. Analyses were limited to
teeth to avoid tissue-specific signatures. We found DNA methylation
patterns within gene bodies generally more similar among
pairs of Neolithic individuals than among Neolithic-Mesolithic
pairs (Figure 2B). However, the Neolithic female (Stuttgart) was
found closer to one Mesolithic male (Loschbour) prepared in
the same laboratory than to the individual 2H10 prepared in
our laboratory. This indicated technical batch effects impacting
DNA methylation inference. To avoid these effects and sex-specific
signatures, we further restricted the analyses to those male
individuals characterized to highest coverage in different laboratories.
We identified a total of 611 gene promoters in which DNA
methylation shifted by at least 50% on average in both Neolithic
individuals (Jerpoint1412 and 2H11) relative to the Mesolithic individual
(Loschbour4; Data S3A). Although not significantly enriched
in particular functional categories, these included more instances
undergoing hyper-methylation during the Neolithic than
hypo-methylation (p = 1.6 31015). Examples include C1Qb, the
first chain of the humoral immune complement response,49 and
LCK, a key signaling molecule involved in T cell maturation.50
Further work is required to assess whether such signatures
reflect regulatory changes in dental or blood tissues.
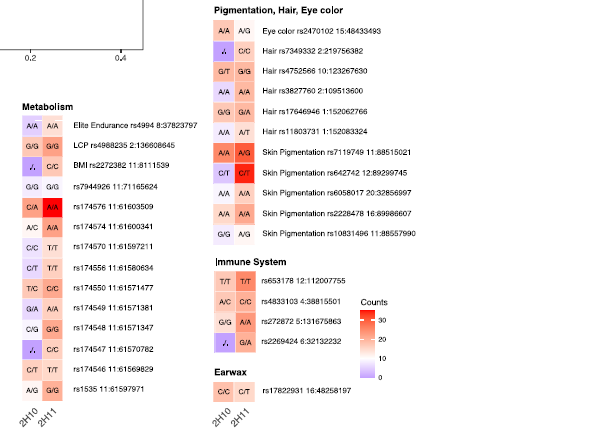
We next attempted to predict the age at death of the two individuals
sequenced to high coverage following a previously
reported approach,51 leveraging DNA methylation levels inferred
within 1 kb around 27,528 CpG positions forming the Illumina 27k
methylation Bead Chip system. Although significantly correlated,
the DNA methylation values returned by DamMet48 were
consistently lower than those measured on a normalization
gold standard panel used for epigenetic age inference (Data
S3B).52 This precluded using the DNAmAge methylation clock
predictor to precisely estimate age at death. The latter was
instead estimated using DNA methylation levels at 11 clock-
CpGs known to undergo DNA methylation changes in teeth
with age.53 Despite cross-validation rates of 0.897–0.939 on
modern DNA data, this methodology showed limited precision
with ancient DNA data and provided confidence intervals spanning
up to 46 years (Data S3C). Pending necessary methodological
improvements, this approach suggested that 2H10 potentially
died younger than 2H11 (29 years old versus 42 years old).
Although precise phenotypic inference was not possible using
DNA methylation patterns, we used the genotypes measured for
the two high-coverage individuals at 90 loci to infer morphological,
pigmentation, behavioral, and medical phenotypes (Figure
2C; Data S2B). Both individuals were lactose intolerant and
were not carriers of the increased risk allele for the celiac disease
at rs653178, for increased addiction behavior at rs1800497 and
rs2283265, or for elite endurance performance at rs4994. They
likely had brown eyes and dark hair (prediction probabilities =
0.844–0.997) but did not carry alleles showing additive effects
on hair thickness (rs4752566 and rs3827760). Individual 2H11
was most likely dark skinned (prediction probability = 0.960;
Data S2B), although 2H10 did not have a (very) pale but intermediate
to dark skin. Interestingly, the two individuals carried
different haplotypes at the mono-amine oxidase A locus, which
encodes for an enzyme involved in the oxidation of dopamine,
norepinephrine, and serotonin neurotransmitters.54 These entail
different combinations of alleles associated with higher (e.g.,
rs3027407 and rs909525) or lower (e.g., rs1137070,
rs1465107, rs2072743, and rs6323) enzymatic production.
This, and the existence of gene-environment interactions,54
make the exact phenotypic consequences difficult to predict.
However, the genetic differences observed at this locus suggest
different risks of developing aggressive and impulsive behavior
in these individuals.55
Genetically identified as female, individual 1H14 could thus not
be tested with this approach. Sequence data displayed post
mortem DNA degradation characteristic of DNA extracts partially
treated with USER37 (Figure S1A), and genetic affinities were in
line with those Mont-Aime males showing minimal contamination.
As mtDNA data do not necessarily reflect autosomal
contamination rates,38 individual 1H14 was included in downstream
analyses. Overall, we report a total of 24 ancient genomes
at 0.20- to 23.88-fold average depth of coverage (median
= 0.96-fold), including two at high depth (2H10 and 2H11,
13.91- and 23.88-fold, respectively). Supporting our data quality,
error rates ranged between 0.057% and 0.083% substitutions
per base (median = 0.069%), which is approximately half the
average error rate estimated in ancient (high-coverage) genomes
previously reported (Figures S1C–S1E).
Post mortem DNA decay, especially cytosine deamination, is
known to have a differential impact on methylated and unmethylated
CpGs.46 This provides an opportunity to map DNA methylation
marks on ancient genomes, assuming USER treatment of
DNA extracts47 and sufficient sequencing efforts.48 We leveraged
the two high-coverage genomes characterized here and
four others previously reported4,12 to identify genes that may
have experienced important DNA methylation changes during
the Mesolithic to Neolithic transition. Analyses were limited to
teeth to avoid tissue-specific signatures. We found DNA methylation
patterns within gene bodies generally more similar among
pairs of Neolithic individuals than among Neolithic-Mesolithic
pairs (Figure 2B). However, the Neolithic female (Stuttgart) was
found closer to one Mesolithic male (Loschbour) prepared in
the same laboratory than to the individual 2H10 prepared in
our laboratory. This indicated technical batch effects impacting
DNA methylation inference. To avoid these effects and sex-specific
signatures, we further restricted the analyses to those male
individuals characterized to highest coverage in different laboratories.
We identified a total of 611 gene promoters in which DNA
methylation shifted by at least 50% on average in both Neolithic
individuals (Jerpoint1412 and 2H11) relative to the Mesolithic individual
(Loschbour4; Data S3A). Although not significantly enriched
in particular functional categories, these included more instances
undergoing hyper-methylation during the Neolithic than
hypo-methylation (p = 1.6 31015). Examples include C1Qb, the
first chain of the humoral immune complement response,49 and
LCK, a key signaling molecule involved in T cell maturation.50
Further work is required to assess whether such signatures
reflect regulatory changes in dental or blood tissues.
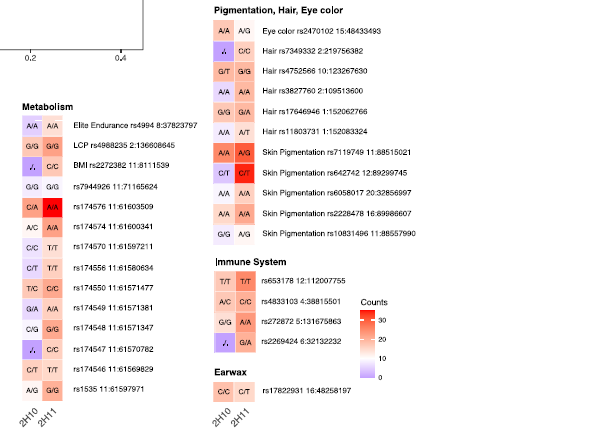
We next attempted to predict the age at death of the two individuals
sequenced to high coverage following a previously
reported approach,51 leveraging DNA methylation levels inferred
within 1 kb around 27,528 CpG positions forming the Illumina 27k
methylation Bead Chip system. Although significantly correlated,
the DNA methylation values returned by DamMet48 were
consistently lower than those measured on a normalization
gold standard panel used for epigenetic age inference (Data
S3B).52 This precluded using the DNAmAge methylation clock
predictor to precisely estimate age at death. The latter was
instead estimated using DNA methylation levels at 11 clock-
CpGs known to undergo DNA methylation changes in teeth
with age.53 Despite cross-validation rates of 0.897–0.939 on
modern DNA data, this methodology showed limited precision
with ancient DNA data and provided confidence intervals spanning
up to 46 years (Data S3C). Pending necessary methodological
improvements, this approach suggested that 2H10 potentially
died younger than 2H11 (29 years old versus 42 years old).
Although precise phenotypic inference was not possible using
DNA methylation patterns, we used the genotypes measured for
the two high-coverage individuals at 90 loci to infer morphological,
pigmentation, behavioral, and medical phenotypes (Figure
2C; Data S2B). Both individuals were lactose intolerant and
were not carriers of the increased risk allele for the celiac disease
at rs653178, for increased addiction behavior at rs1800497 and
rs2283265, or for elite endurance performance at rs4994. They
likely had brown eyes and dark hair (prediction probabilities =
0.844–0.997) but did not carry alleles showing additive effects
on hair thickness (rs4752566 and rs3827760). Individual 2H11
was most likely dark skinned (prediction probability = 0.960;
Data S2B), although 2H10 did not have a (very) pale but intermediate
to dark skin. Interestingly, the two individuals carried
different haplotypes at the mono-amine oxidase A locus, which
encodes for an enzyme involved in the oxidation of dopamine,
norepinephrine, and serotonin neurotransmitters.54 These entail
different combinations of alleles associated with higher (e.g.,
rs3027407 and rs909525) or lower (e.g., rs1137070,
rs1465107, rs2072743, and rs6323) enzymatic production.
This, and the existence of gene-environment interactions,54
make the exact phenotypic consequences difficult to predict.
However, the genetic differences observed at this locus suggest
different risks of developing aggressive and impulsive behavior
in these individuals.55
Genetically identified as female, individual 1H14 could thus not
be tested with this approach. Sequence data displayed post
mortem DNA degradation characteristic of DNA extracts partially
treated with USER37 (Figure S1A), and genetic affinities were in
line with those Mont-Aime males showing minimal contamination.
As mtDNA data do not necessarily reflect autosomal
contamination rates,38 individual 1H14 was included in downstream
analyses. Overall, we report a total of 24 ancient genomes
at 0.20- to 23.88-fold average depth of coverage (median
= 0.96-fold), including two at high depth (2H10 and 2H11,
13.91- and 23.88-fold, respectively). Supporting our data quality,
error rates ranged between 0.057% and 0.083% substitutions
per base (median = 0.069%), which is approximately half the
average error rate estimated in ancient (high-coverage) genomes
previously reported (Figures S1C–S1E).