|
Post by Admin on Sept 19, 2019 17:40:45 GMT
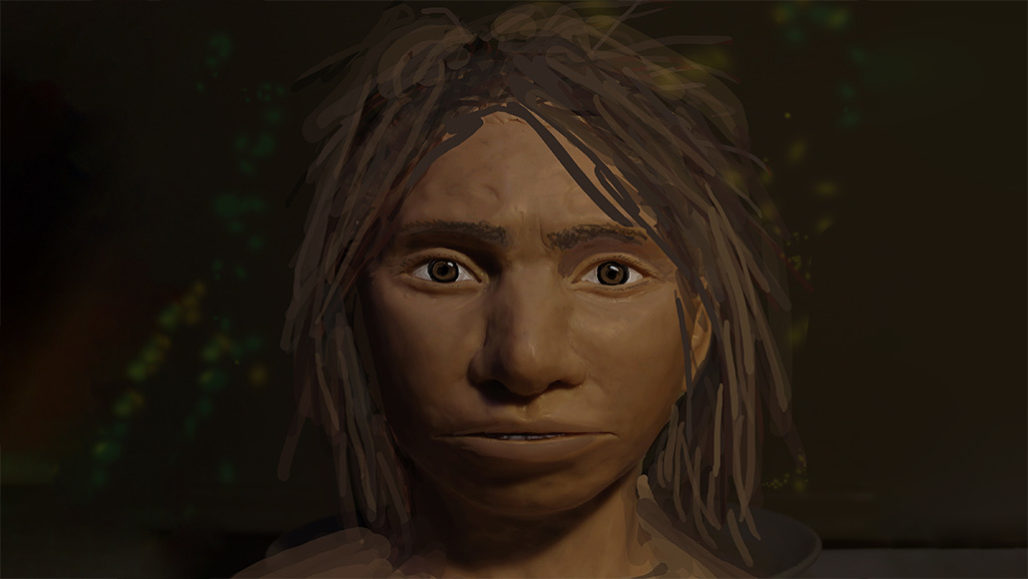 Scientists have painted a portrait of a young female who belonged to a mysterious, humanlike population known as Denisovans around 50,000 years ago. Here’s the kicker: Only a handful of Denisovan fossils have been found, including the youngster’s pinky finger. So a team led by evolutionary geneticists David Gokhman and Liran Carmel of the Hebrew University of Jerusalem reconstructed the Denisovan teen’s skeleton using only a palette of ancient DNA patterns. A description of how the researchers transformed DNA into a physical appearance appears September 19 in Cell. “This is the first reconstruction of the skeletal anatomy of Denisovans,” Carmel says.  A drawing based on that skeleton shows the Denisovan gazing ahead coolly with wide, dark eyes framing the bridge of a broad nose. That profile, and the rest of the girl’s appearance, was gleaned from key changes to parts of her DNA that regulate the activity of genes involved in skeletal development, the team says. Summary Denisovans are an extinct group of humans whose morphology remains unknown. Here, we present a method for reconstructing skeletal morphology using DNA methylation patterns. Our method is based on linking unidirectional methylation changes to loss-of-function phenotypes. We tested performance by reconstructing Neanderthal and chimpanzee skeletal morphologies and obtained >85% precision in identifying divergent traits. We then applied this method to the Denisovan and offer a putative morphological profile. We suggest that Denisovans likely shared with Neanderthals traits such as an elongated face and a wide pelvis. We also identify Denisovan-derived changes, such as an increased dental arch and lateral cranial expansion. Our predictions match the only morphologically informative Denisovan bone to date, as well as the Xuchang skull, which was suggested by some to be a Denisovan. We conclude that DNA methylation can be used to reconstruct anatomical features, including some that do not survive in the fossil record. 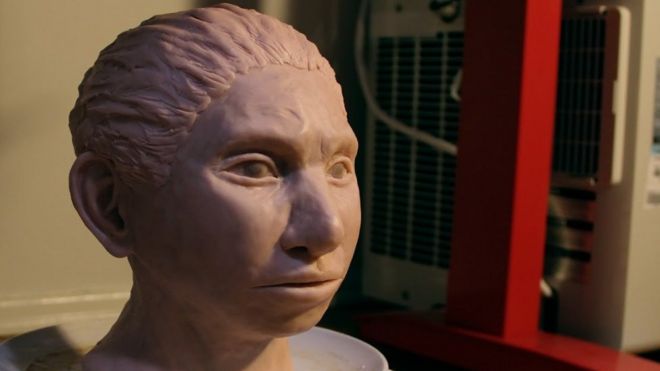 Very little is known about the anatomy of Denisovans. The first specimen, Denisova 3, comprises a manual phalanx found in the Denisova cave in Siberia, dated between 74 and 82 thousand years ago (kya) (Krause et al., 2010). DNA extracted from this bone indicated that this individual belonged to a sister group of Neanderthals (Meyer et al., 2012), thereafter called Denisovans. These two groups separated 390–440 kya (Prüfer et al., 2017), and their ancestors split from our lineage between 520 and 630 kya (Prüfer et al., 2017), though these datings are still under debate (Mafessoni and Prüfer, 2017, Rogers et al., 2017). Based on this genome, Denisovan ancestry of up to 6% was detected in present-day Melanesians and Aboriginal Australians and to a lesser level in East Asians, Native Americans, and Polynesians (Meyer et al., 2012, Prüfer et al., 2014, Racimo et al., 2015, Reich et al., 2010, Skoglund and Jakobsson, 2011). Some introgressed Denisovan haplotypes might have conferred modern humans (MHs) an adaptive advantage in high-altitude (Beall et al., 2010) and cold climates (Racimo et al., 2017). Despite our growing understanding of their genetics, findings that provide information on Denisovan anatomy remain scarce. The only confirmed Denisovan samples hitherto are the aforementioned Denisova 3 phalanx, from which a 30x genome was sequenced (Meyer et al., 2012), a lower jawbone (Chen et al., 2019), and several teeth (Chen et al., 2019, Sawyer et al., 2015, Slon et al., 2017). Anatomical studies of the teeth revealed that the Denisovan molars differ in their cusp and root morphology, and their size is outside the range of MHs and mostly outside the range of Neanderthals too (Chen et al., 2019, Sawyer et al., 2015, Slon et al., 2017). The jawbone was shown to be robust, protruding, with a long dental arcade and no chin (Chen et al., 2019). Studying differences in anatomy between human groups is critical in understanding human-specific adaptations, selective pressures, and developmental trajectories as well as the phenotypic effects of introgression events. 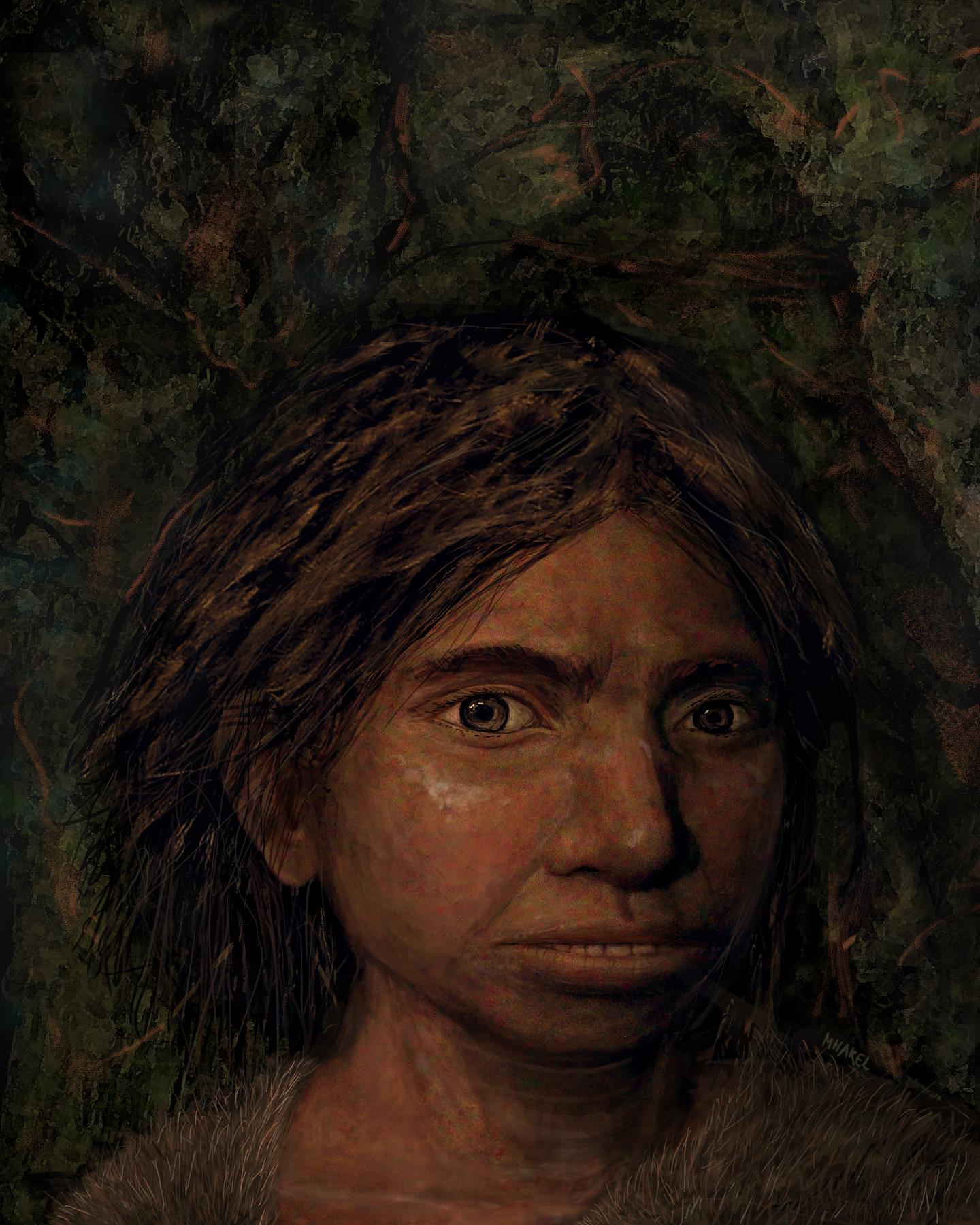 While the Denisovan DNA sequence potentially bears ample information on its anatomical features, our current ability to decode these data is very restricted. A direct approach is to examine the biological consequences of substitutions that alter protein sequence. However, less than 100 fixed nonsynonymous substitutions distinguish MHs from the Denisovan and Neanderthal, whereas the remaining ∼30,000 fixed changes are noncoding or synonymous (Prüfer et al., 2014). Although many of the noncoding changes are likely neutral (or nearly so), many others probably alter gene activity and may be highly informative to anatomy. However, pinpointing such variants is notoriously difficult. A possible approach to circumvent this is to predict the combined effect of SNPs that are known to be associated with various traits. Prediction accuracy for traits such as skin, hair, and eye pigmentation exceeds 80% in Europeans (Walsh et al., 2013), but for the vast majority of traits, genome-wide association study (GWAS)-based predictions reach substantially lower accuracy levels (Price et al., 2015), including in facial morphology (Brinkley et al., 2016, Cole et al., 2016, Erlich, 2017, Liu et al., 2012, Shaffer et al., 2016). Moreover, the ability to extrapolate European-based GWASs to non-European populations was shown to be very limited (Martin et al., 2017). Perhaps most importantly, GWASs are based on within-population variability, which usually reflects variants that emerged more recently. However, older variants that separate more deeply diverged lineages and variants with considerable phenotypic effects are more likely to reach fixation and are therefore unlikely to be pinned down in GWASs, even if their effect is substantial (Martin et al., 2017, Price et al., 2015). Together, these factors limit the applicability of GWAS-detected variants in morphological analyses of deeply diverged groups, such as the Denisovan. Ideally, to further the understanding of Denisovan anatomy, one would strive to directly measure gene expression, which is more readily interpretable than noncoding sequence changes. However, RNA molecules rapidly degrade in ancient samples and are unavailable for sequencing. Therefore, we used DNA methylation, a key regulatory layer of the genome, as a proxy for gene activity. Here, we developed a method that compares the Denisovan DNA methylation patterns to those of MHs, Neanderthals, and chimpanzees and infers which genes may have become up- or downregulated along each lineage. Next, we linked these changes to potential phenotypic alterations. We did so by analyzing phenotypes that are known to be incurred by loss-of-function mutations in these genes and could therefore be roughly paralleled with reduced activity. Importantly, unlike previous efforts to make quantitative morphological estimations (Claes et al., 2014, Erlich, 2017, Lippert et al., 2017), our aim was considerably more modest; we strove to reconstruct a qualitative skeletal profile by predicting traits that are divergent between the human groups and—when possible—to determine their direction of change. Our rationale was that providing accurate magnitudes of anatomical changes is infeasible mainly because precise activity levels of archaic human genes cannot be determined, and even in present-day samples, the quantitative contribution of each gene to the trait is currently impossible to predict. We quantified the accuracy of our method by applying it to the Neanderthal and the chimpanzee and then comparing our predictions with the known morphology of these groups. We show that we reach prediction precision of 82.8% in reconstructing traits that separate Neanderthals and MHs and 87.9% in predicting their direction of change. In the chimpanzee, we reach a similar performance, with 90.5% precision at predicting which traits are divergent and 90.9% in predicting their direction of change. By applying our method to the Denisovan, we propose a methylation-based profile of Denisovan morphology.
|
|
|
Post by Admin on Sept 20, 2019 17:26:50 GMT
Using Changes in DNA Methylation for Phenotypic Reconstruction We have previously developed a method to reconstruct full DNA methylation maps of ancient genomes (Gokhman et al., 2014). This method is based on the analysis of damage patterns in ancient DNA, whereby pre-mortem methylated and unmethylated cytosines leave distinct signatures that can be used to differentiate between them (Briggs et al., 2010, Gokhman et al., 2014, Pedersen et al., 2014). Using this method, we reconstructed the methylomes of Denisova 3, two Neanderthals (Altai and Vindija), and five anatomically modern humans from 45 to 7.5 kya (Gokhman et al., 2014, Gokhman et al., 2017a). Together with bone methylomes from 55 present-day humans and five chimpanzees, we were able to chart a comprehensive map of differentially methylated regions (DMRs) that emerged along the different branches of the hominin tree (Gokhman et al., 2017a). To be able to reconstruct the morphology of an archaic individual using these DMRs, three nested conditions should be met: (1) the DMRs should reflect lineage-specific changes, rather than within-lineage variation that is driven by factors such as age, sex, or bone type; (2) lineage-specific methylation changes should reflect changes in gene activity levels; and (3) changes in gene activity should be associated with known phenotypic effects. The first condition was accounted for during the DMR calling phase, where we harnessed the diversity across the 68 modern human, Neanderthal, Denisovan, and chimpanzee samples to filter out bone-type-, age-, disease- and sex-specific DMRs. This was achieved by examining the methylation levels of each DMR and requiring that all samples in a human lineage cluster outside all samples from the other lineages, regardless of age, sex, disease condition, or bone type. In other words, loci whose methylation levels differ across skeletal parts, age, health state, or sex were removed; see full details in Gokhman et al. [2017a]. Importantly, the fact that these DMRs have similar methylation levels across various skeletal regions of the same human group suggests that they are not constrained to a specific bone type but rather exist throughout the skeleton, including in the cranium, limbs, and teeth (Gokhman et al., 2016, Gokhman et al., 2017a).  Figure S1 Unidirectional Promoter Methylation Changes Are Predictive of Chimpanzee Anatomy, Related to Figures 1 and 3 and STAR Methods, Validation and Accuracy Estimation To focus on DMRs reflecting the most extensive methylation changes, we also required that DMRs represent at least a 50% change in methylation (for example, from a regional average of 30% methylation to a regional average of 80% methylation) and span a minimum of 50 CpG positions. This filter also removes most environmentally induced DMRs, as their effect size rarely exceeds 10% methylation change (Gokhman et al., 2017b). Therefore, our list of DMRs is likely to capture marked pan-skeletal evolutionary changes between the human lineages and to be devoid of regions that show within-lineage variability (Figure S1A). These strict filters and the use of over 60 samples are expected to considerably reduce false discoveries at the expense of leaving more subtle lineage-specific methylation changes undetected. Moreover, we conjecture that such substantial changes between lineages are more likely to result in observable phenotypes. The final list of lineage-specific DMRs includes 873 MH-derived DMRs, 939 archaic-derived DMRs (i.e., DMRs that emerged in the ancestors of Neanderthals and Denisovans), 570 Neanderthal-derived DMRs, 443 Denisovan-derived DMRs, and 2,031 DMRs that separate chimpanzees from all human groups (Figure S1B). Importantly, most gene-disease associations at the basis of HPO come from works that linked protein sequence, rather than regulatory mutations, to diseases. Many of these mutations result in loss of function, where one or both copies of a gene are dysfunctional. Examples of such mutations include frameshifts, gain of stop codons, or complete gene deletions (Hamosh et al., 2005, Li et al., 2015, MacArthur et al., 2012). Loss-of-function mutations are sometimes counterbalanced by upregulation of the other gene copy (El-Brolosy and Stainier, 2017). However, this is unlikely to be the case in HPO phenotypes, as their underlying mutations were shown to result in an observable phenotype, suggesting that their loss of function is not fully compensated. Therefore, the loss of function that underlies most phenotypes in HPO could be paralleled to a partial or complete decrease in gene activity. Although the exact level of decrease associated with hypermethylation is impossible to evaluate, these phenotypes are key to understanding the direction of phenotypic change when these genes show reduced activity in humans. We use this logic to associate the phenotypes reported in HPO with the hominin group exhibiting the gene-silencing patterns (Figure 1). For all DMGs eventually used for the Denisovan skeletal reconstruction, we have validated the assertion that the observed phenotype is a result of loss of function. We have also asserted that most phenotypes are known to be driven by heterozygous mutations, suggesting that even partial loss of function results in a phenotype (see STAR Methods). HPO also contains information on the penetrance of each phenotype, dividing them into frequent and non-frequent based on whether they appear in >50% of patients or not (Köhler et al., 2014). Here, we used only frequent HPO phenotypes.  Figure 1 The Pipeline of Reconstructing Denisovan Anatomy Using DNA Methylation Changes The DNA methylation maps that we use came from bones and teeth. Hence, linking DMRs to expression changes would be most reliable in these tissues. We have therefore used Gene ORGANizer—a database linking genes to the organs they phenotypically affect (Gokhman et al., 2017c)—to further filter the list of phenotypes and discard genes that are unknown to affect bones or teeth. This left a total of 597 skeletal DMGs on the hominin or chimpanzee lineages, linked to 1,528 skeleton-related HPO phenotypes (Table S2). Filtering for Unidirectionality We divided all phenotypes into two groups: directional and non-directional. Directional phenotypes were defined as those that could be described along a one-dimensional axis of change, such as higher-lower, accelerated-delayed, etc. Examples of such phenotypes include Delayed skeletal maturation (HP:0002750) and Biparietal narrowing (HP:0004422). Non-directional phenotypes are phenotypes that could not be described on a one-dimensional scale, such as Abnormality of the face (HP:0000271), and Dental malocclusion (HP:0000689). Here, we strove to predict the direction of morphological changes. Therefore, non-directional phenotypes were discarded from our analysis, leaving 815 skeleton-related directional HPO phenotypes, each linked to the gene or genes that have been shown to underlie the phenotype (Table S2). We then intersected this list with the skeletal DMGs and determined an expected direction of change in expression (hyperactive or hypoactive) in each hominin relative to MHs (Figure 1; Table S3). We make a distinction between two types of predictions: predictions of trait divergence (e.g., finger length differs between MHs and Neanderthals) and predictions of trait divergence where a direction of change could be assigned as well (e.g., the fingers are longer in MHs compared to Neanderthals). One can think of each promoter DMR that is linked to an HPO phenotype as a predictor of the direction of divergence of that phenotype. If all predictors that relate to the same trait pointed to the same direction (hereinafter, unidirectionality), we make a directional prediction to this trait. However, if different predictors provided contradicting conclusions regarding the direction of change, we cannot ascertain a direction of change and only predict that the trait is divergent (Figures 2A and S2A). Theoretically, such opposing directions of change could point to no net phenotypic change or to neutral evolution. However, this is less likely in DMRs that are in promoters and show a large methylation change. Overlapping predictions (e.g., Short iliac bones and Hypoplastic ilia) were grouped together and counted as one trait prediction (see STAR Methods).
|
|
|
Post by Admin on Sept 20, 2019 21:46:57 GMT
 Figure 2 Unidirectional Promoter Methylation Changes Are Predictive of Neanderthal Anatomy (A) An example of a unidirectional trait. See Figure S2A for an example of a non-unidirectional trait. The DMRs column shows promoter DMRs along the different lineages (N, Neanderthal; D, Denisovan; M, modern human). Up and down arrows mark hyper- and hypomethylation, respectively. The DMGs column shows predicted gene activity change for each of the DMGs. Increased or decreased activity is marked with up or down arrows, respectively. All five genes show patterns of decreased activity in MHs compared to Neanderthals. The HPO phenotypes column shows phenotypes associated with each of the genes. All five phenotypes associated with the genes point to reduced pelvic size in MHs compared to Neanderthals. (B) The 17 divergent skull phenotypes for which a direction of change could be assigned. See Figure S2B for non-craniofacial phenotypes. Whenever overlapping phenotypes were merged, the displayed HPO ID belonged to the most general phenotype. Each prediction was tested against known Neanderthal morphology. Checkmarks represent correct predictions (trait is divergent and was predicted in the direction that matches known morphology); X marks predictions where the known Neanderthal phenotype is opposite to the prediction.  Figure S2 Unidirectional Promoter Methylation Changes Are Predictive of Neanderthal Anatomy, Related to Figure 2 and STAR Methods, Unidirectionality Filtering A. An example of a trait where promoter methylation changes point to divergence, but the direction of change could not be determined. See Figure 2A for an example of a unidirectional trait. The DMRs column shows promoter DMRs along the different lineages (N – Neanderthal, D – Denisovan, M – MH). Up/down arrows mark hyper/hypomethylation, respectively. The DMGs column shows predicted gene activity change for each of the DMGs. Increased/decreased activity is marked with up/down arrows, respectively. All three genes show patterns of decreased activity in MHs compared to Neanderthals. The HPO phenotypes column shows phenotypes associated with each of the genes. These phenotypes are contradicting, suggesting that suborbital ridges are divergent between MHs and Neanderthals, but the direction of change cannot be determined. B. The 16 non-craniofacial divergent phenotypes for which a direction of change could be assigned. See Figure 2B for skull phenotypes. Whenever overlapping phenotypes were merged, the displayed HPO ID belongs to the most general phenotype. Check marks represent correct predictions (trait is divergent and was predicted in the direction that matches known morphology), X marks predictions where the known Neanderthal phenotype is opposite to the prediction. Unidirectionality filtering was applied in three nested levels: (1) on DMRs within the same gene promoter, (2) on DMGs linked to the same HPO phenotype, and (3) on HPO phenotypes linked to the same trait (Figures 1 and S3). For example, there are four DMGs associated with the HPO phenotype High forehead (HP:0000348): LETM1 and SOX9, whose promoter became hypermethylated along the MH lineage, and FGFR3 and NELFA, whose promoter became hypomethylated in archaic humans. Thus, it passed the unidirectionality filter as all four DMRs point to lower gene activity in MHs and, thus, probably a higher forehead (as indeed observed in MHs compared to Neanderthals [Weaver, 2009]). In cases where the DMRs point to different directions, we predict that the trait is divergent but do not predict a direction of change. As demonstrated later, a focus on unidirectional predictions reduces the number of predictions but at the same time increases prediction strength. Moreover, this filtering is likely to enrich for traits that are under selection, as selection is expected to drive changes in gene activity in the same direction (Fraser et al., 2010).
|
|
|
Post by Admin on Sept 21, 2019 7:15:57 GMT
 Figure S3 Descriptive Statistics of DMRs, DMGs, and HPO phenotypes, Related to STAR Methods, Unidirectionality Filtering In order to estimate the performance of our approach, we applied it to two groups for which we have extensive skeletal information: the Neanderthal and the chimpanzee. For each group, we evaluated two measures of performance: precision (PRE), defined as the fraction of true predictions from the total number of predictions, and true positive rate, or sensitivity (SEN), defined as the fraction of all known divergent traits that we correctly predicted. As mentioned above, we made a distinction between two types of predictions: predictions that a trait has diverged between the hominin groups (divergence) and predictions of the direction of change (direction). Focusing first on the Neanderthal, we predicted 64 skeletal traits where Neanderthals are expected to differ from MHs (Table S4). We defined a prediction to be correct if the trait was previously shown to be divergent between Neanderthal and MH skeletons. To this end, we assembled a list of 107 known Neanderthal-MH differences (Table S5; see STAR Methods), including traits whose phenotypic distributions partly overlap between the groups. Of these divergent traits, 75 had at least one corresponding HPO phenotype and could therefore be compared against our predictions. Out of our 64 predictions, 53 are indeed known to be divergent between Neanderthals and MHs, giving PREdivergence = 82.8%. Of the 53 correctly predicted traits, 33 passed all three unidirectionality filters and were thus assigned a direction of change as well. Out of these 33 traits, we correctly predicted the direction of 29, giving PREdirection of 87.9% (Figures 2B, S2B, and 3A; see Quantification and Statistical Analysis).  Figure 3 Precision and Sensitivity of Neanderthal Skeletal Reconstruction To assess the sensitivity of our approach, which measures how many of the known Neanderthal traits we are able to detect using DNA methylation, we analyzed the aforementioned list of traits (Table S5) and examined how many of them were predicted by our method. We identified 62 of the 75 divergent traits, giving SENdivergence of 82.7%. Of the 62 correctly predicted divergent traits, we could assign a direction of change to 46. We correctly predicted the direction of 36 out of these 46 traits, giving SENdirection of 78.3% (Figure 3B). Correct predictions do not necessarily reflect high accuracy but could rather stem from elevated probability to detect divergent traits by chance. Thus, in order to further evaluate the performance of our approach, we compared its precision and sensitivity to random expectation (see STAR Methods). For precision, the random expectation of PREdirection is 0.5, as each trait has an equal probability to change in one direction or the opposite. To assess the random expectation of PREdivergence, we annotated all 813 directional skeletal terms on HPO. For 644 of them (79.0%), we were able to determine whether they differ between Neanderthals and MHs, based on previous comparative analyses of Neanderthal morphology (Tables S2 and S5). The rest were discarded due to insufficient information in the fossil record (e.g., Delayed calcaneal ossification, HP:0008142), ambiguity (e.g., Skeletal dysplasia, HP:0002652), or contradicting evidence (e.g., Long phalanx of finger, HP:0006155, where Neanderthals have shorter proximal, but longer intermediate and distal, phalanges [Klenerman and Wood, 2006]). We found that 361 of the 644 HPO phenotypes differ between Neanderthals and MHs, giving a random expected value of PREdivergence of 56.1%. Interestingly, the number of divergent phenotypes varies considerably across anatomical regions. For example, 81.0% of facial phenotypes differ between the groups, suggesting that the facial region is particularly divergent (p = 2.8 × 10−5, hypergeometric test). This was previously proposed based on comparative anatomical studies (Weaver, 2009) as well as DNA methylation patterns (Gokhman et al., 2017a). Our approach performs significantly better than random expectation, both in predicting divergent traits (PREdivergence = 82.8% compared to 56.1%, 1.48x, p = 1.5 × 10−6, hypergeometric test) and in predicting the direction of change (PREdirection = 87.9% compared to 50%, 1.76x, p = 5.5 × 10−6, Figure 3A). We repeated the above analysis while dividing the phenotypes into several skeletal regions. In all cases, our prediction power is significantly higher than random expectation (Figure 3C). To assess the contribution of the different filters to the overall performance of our method, we tested four subsets of DMRs with increasing filtering stringency. Each subset of DMRs added a filtering step to the previous subset: fixation, overlap with promoters, and unidirectionality. For each subset of DMRs, we examined its ability to predict Neanderthal skeletal features. We found that the probability of any DMR to be linked to a divergent trait is only 2.3% higher than expected by chance (p = 0.3, hypergeometric test). However, this probability increases with every filtering step, reaching +68.3% for non-variable promoter DMRs that passed the unidirectionality filtering (p = 9.5 × 10−3, Figure 3D). Thus, we conclude that prediction power significantly improves by (1) focusing on fixed or nearly fixed DMRs, (2) focusing on promoter DMRs, and (3) applying unidirectionality filters. We have also lowered the stringency of our DMR-detection method in order to study how this affects prediction accuracy. We found that while relaxing the criteria for DMR-detection slightly reduces performance, it is still significantly higher than expected by chance (see STAR Methods; Table S1). To further examine the robustness of our approach, we applied it on chimpanzees, which are ∼10x more deeply diverged from MHs compared to Neanderthals. We reached similar precision levels to the ones obtained in the Neanderthal reconstruction: 42 traits were predicted to differ between the groups, of which 38 are indeed known to separate humans and chimpanzees (PREdivergence = 90.5% compared to random expectation of 75.0%, 1.21x, p = 9.0 × 10−3, hypergeometric test). 22 of the traits passed all three unidirectionality filters, and thus, we were also able to predict their direction. In 20 of them, the prediction was correct (PREdirection = 90.9% compared to random expectation of 50%, 1.82x, p = 6.1 × 10−5). The fraction of known chimpanzee traits that we are able to predict (i.e., sensitivity) is lower compared to the Neanderthal: SENdivergence = 61.4%, 1.15x, p = 0.08; SENdirection = 71.9%, 1.59x, p = 0.04; Table S4; Figures S1C–S1E), as expected due to the deeper divergence time of chimpanzees. Finally, to examine the performance of our method when applied to other types of data, we tested it on enhancer marks as well as expression changes separating humans and chimps (Prescott et al., 2015). Here too, we reach prediction levels significantly higher than expected by chance, particularly for the expression data (PREdivergence = 87.5%, p = 0.039; PREdirection = 76.9%, 1.54x, p = 0.047; Table S6; see STAR Methods). To compare between the prediction power of methylation and expression, we down-sampled our DMR list to resemble the number used to produce the aforementioned expression data. With a similar number of samples, expression-based analysis provides better predictions of divergent traits than methylation-based analysis (87.5% compared to 75.4%, see STAR Methods). This is unsurprising given that in our method methylation serves as a proxy for expression. In summary, using three steps—(1) linking promoter methylation changes to downregulation, (2) associating these changes with phenotypes of loss-of-function mutations, and (3) applying unidirectionality filtering—we are able to reconstruct phenotypic profiles with ∼87% precision and ∼73% sensitivity. For about half of the traits, we are able to predict the direction of change, with ∼89% precision and ∼76% sensitivity (Figures 2, 3, S1, and S2; Table S4).
|
|
|
Post by Admin on Sept 21, 2019 19:35:43 GMT
Reconstructing Denisovan Anatomy Given the precision and sensitivity demonstrated by our approach, we turned to apply our method to the Denisovan. Denisovan traits can be divided into two classes: traits where the Neanderthal and Denisovan both differ from MHs and traits where the Denisovan differs from the Neanderthal. The former class represents traits that have likely evolved along the MH lineage or in the ancestors of Neanderthals and Denisovans, while the latter represents traits that have likely evolved along one of the archaic human branches. Given the number of DMRs identified along each branch and the phylogenetic architecture (Figure S1B), most traits are expected to fall into the first category. These are also expected to be the most reliable predictions, as they are based on DMRs that are fixed across all 60 MH samples and where methylation patterns differ between MHs and archaic humans throughout the skeleton, including the skull (Gokhman et al., 2017a). To enhance our prediction accuracy for this class, we took only traits where the prediction for the Neanderthal was correct; i.e., we considered only DMGs whose methylation patterns (which the Denisovan and Neanderthal share) were shown to be predictive of the phenotypic change. We also conducted a comparison of the Denisovan to the Neanderthal using Denisovan-derived and Neanderthal-derived DMGs to identify traits in which the two hominins differ from one another. Overall, we identified 56 traits in which the Denisovan is expected to be different from MHs or Neanderthals, and in 32, we were able to predict a direction of change (Table S7). As the method is based on associating HPO phenotypes with the hominin group exhibiting downregulation patterns, we verified that all HPO phenotypes used in the analysis are indeed a result of loss-of-function mutations in human patients (Hamosh et al., 2005) (Table S3). The 32 unidirectional traits allowed us to suggest a reconstruction of Denisovan skeletal features (Figures 4 and 5). As expected, most Denisovan traits (21 out of 32) are predicted to be shared with the Neanderthal. The 21 shared traits include characteristic Neanderthal features such as robust jaws, low cranium, increased cranial base growth, low forehead, thick enamel, wide pelvis, large femoral articulations, wide fingertips, and large ribcage (Aiello and Dean, 2002, Weaver, 2009). The other 11 unidirectional traits are based on DMGs with distinct Denisovan or Neanderthal evolution and therefore represent morphological aspects where the two archaic humans are expected to differ from one another. These 11 traits can be divided into three groups according to the branches in which their associated DMGs emerged. The first group includes changes that emerged along the Denisovan lineage, i.e., traits where Denisovan features are expected to differ from both MHs and Neanderthals, and includes three morphologies: (1) elongated dental arch, (2) enlarged mandibular condyle (the posterior protuberance which is part of the mandibular joint), and (3) biparietal expansion, i.e., increased distance between the parietal bones of the cranium. The second group includes Neanderthal-specific changes and therefore represents traits where the Denisovan is expected to resemble MHs rather than Neanderthals. This group includes two morphologies: (1) broad temporal bones compared to the width of the anterior mandible and (2) premature loss of permanent teeth. The last group includes six changes where several of their underlying DMGs emerged along the MH or archaic branches and others emerged in the Neanderthal. In these traits, the three hominin groups are expected to differ from one another. These traits are bone mineral density, face width, metaphyseal and diaphyseal width, facial protrusion (prognathism), scapular size, and skeletal maturation timing (Figures 4 and 5).  Figure 4 Reconstructed Profile of the Denisovan Skull Unlike the Neanderthal skeletal profile, which can be matched against fossil evidence, the Denisovan profile can only be validated at this point with regard to the mandible and teeth. When this manuscript was in the process of peer review, the first confirmed Denisovan jawbone was reported (Chen et al., 2019). This jawbone presents a unique opportunity to test the accuracy of our predictions. We provided four predictions for the mandibular region: (1) high anterior mandible, (2) wide anterior mandible, (3) mandibular protrusion, and (4) long dental arch (Figure 4). Each of these predictions can be tested on the Denisovan mandible twice: once against MHs and once against Neanderthals. We found that seven out of eight predictions match the morphological description of the Denisovan jawbone—the authors report high anterior versus posterior mandible, a very wide anterior mandible, mandibular protrusion, and a long dental arch (Chen et al., 2019). Our profile did not match the reported mandible in the anterior width when compared to Neanderthals—we predicted it to be similar to Neanderthals, whereas the authors report an anterior mandible that is considerably longer than that of Neanderthals (see STAR Methods). Discussion Interestingly, many of the Denisovan traits we reconstruct were identified in Middle and Late Pleistocene fossils from China. These fossils display various Neanderthal-like characteristics, but their phylogenetic classification remains undetermined (Bae, 2010, Li et al., 2017). Probably the most Neanderthal-like are the 100,000- to 130,000-year-old crania from Xuchang, eastern China. The similarity of these crania to those of Neanderthals, together with their eastern geographical location, raise the possibility that they might belong to Denisovans. However, without DNA, this could not be confirmed. The bones include the skull cap and base, but not the face or the jaws, and exhibit the following ten directional morphologies: (1) lateral expansion of the temporal bones; (2) low cranial vault; (3) lateral expansion of the parietal bones, outside the range of Neanderthals and MHs (Suzuki and Takai, 1970); (4) wide cranial base; (5) cranial gracility; (6) prominent supraorbital tori; (7) reduced thickening (restricted nuchal torus) of the occipital bone; (8) sagittal flatness; (9) short inward-sloping mastoid process; and (10) small anterior semicircular canal radii and more superior lateral versus posterior canals. Traits 1–8 have equivalent phenotypes on HPO and could thus be examined against our reconstructed profile. Strikingly, seven of them were identified as divergent traits in our reconstructed Denisovan profile (Figure 4; Table S7). Traits 1–4 are all linked to unidirectional methylation changes, and therefore, their directionality could be predicted too. The directionality we report for all of these traits matches the directionality observed in the Xuchang fossils. Even more outstanding is the fact that we predict no other divergent traits related to the cranial vault (i.e., the region that was preserved in the Xuchang crania) except for the seven that are observed in the Xuchang fossils (Li et al., 2017) (p = 0.035, Figure 4). The almost complete overlap between the Xuchang crania and our reconstructed profile provides the first genetic support to the notion that the Xuchang skulls are related to Denisovans. Denisovan molars are observed to be significantly broader than that of MHs and also mostly outside the range of Neanderthal molars (Chen et al., 2019, Sawyer et al., 2015, Slon et al., 2017). We did not predict larger molars, although this might be related to the fact that, while the HPO database includes some phenotypes that describe changes in tooth size, it does not include phenotypes that are specific to molar size or breadth. However, we do predict longer dental arch, which could potentially be linked to large molars.  Figure 5 Reconstructed Profile of the Denisovan Skeleton The ability to extract phenotypic information from a single gene is limited. Here, we demonstrated that by (1) looking at the set of genes that underlie a trait, (2) considering only marked promoter methylation changes, and (3) adding unidirectionality filters high phenotypic reconstruction accuracy can be attained. Moreover, as our directional predictions pass all three unidirectionality filters, they are likely to represent either higher-level regulatory changes that cascaded to affect several loci (e.g., silencing of a transcription factor that affects the promoter methylation of multiple target genes) or, alternatively, the process of polygenic adaptation, where one direction of phenotypic change is advantageous, thus resulting in coordinated changes in several loci (Fraser et al., 2010). Both options are likely to drive pronounced phenotypic alterations and, therefore, potentially explain why the predictive power increases when looking at the collective effect of unidirectional changes. We summarize the main strengths and weaknesses of the proposed method. (1) Its predictions offer a comparative direction of change, rather than precise quantitative evaluation of the extent of phenotypic change. (2) In instances where there is no unidirectionality, the direction of change cannot be determined. (3) Morphologies that have no equivalent term on HPO cannot be reconstructed. (4) Reconstructed traits that are solely based on Denisovan-specific DMRs (3 predicted traits out of 56 in total) could represent only the Denisova 3 individual, rather than the entire Denisovan population. (5) Due to the strict unidirectionality filtering, and because of the varying number of individuals used for DMR detection along each branch, the method is more accurate at identifying traits that emerged earlier and became fixed or nearly fixed, while ignoring traits with high intra-population variability. At the same time, such unidirectional fixed changes are more likely to be driven by selection (Fraser et al., 2010) and could therefore represent a more interesting subset of morphological alterations. (6) The precision and sensitivity levels we report are partly dependent on the way traits are discretized, as we have clustered overlapping traits together, thus creating morphological units (see STAR Methods). Yet many morphologies could nevertheless be developmentally intertwined, as reflected by the overlapping set of genes that underlie them. (7) Finally, because Denisovan-specific DMRs are based on a single sample, it is yet to be determined which of the Denisovan morphologies we report are confined to Denisovan 3 and which reflect the Denisovan population. In this regard, several observations support the notion that the majority of the reconstructed traits are shared throughout the Denisovan population. (1) In the fossil record, it has been shown that traits that separate a single Neanderthal fossil from MHs tend to be shared by all Neanderthals (Aiello and Dean, 2002). (2) Roughly half of the reconstructed traits are based on DMRs that emerged along the MH lineage. Because such traits are derived in MHs, Denisovans are expected to share the ancestral form of the trait. (3) The entire analysis is based on DMRs that are unaffected by age, sex, or bone type, and therefore, a Denisovan individual from a different age and sex and where the sample was obtained from another bone type is expected to exhibit similar methylation patterns (Gokhman et al., 2017a). It is illuminating that although the reconstruction accuracy levels for the Neanderthal and chimpanzee are very similar, the fraction of known derived traits that have an equivalent HPO phenotype are markedly different. For the Neanderthal, 75 out of 107 derived traits (70%) have a parallel HPO phenotype, whereas for the chimpanzee, where divergence is ∼10x deeper, the fraction is significantly lower (83 out of 201, 41%, p = 1.5 × 10−6, χ2 test). This raises the possibility that along short timescales, the genes that underlie diseases may also be those that underlie evolutionary phenotypic divergence, possibly accounting for the overlap between the two lists. This is also supported by a study that suggested that the loci involved in craniofacial disorders are likely to underlie normal variation too, both within humans and between humans and chimpanzees (Claes et al., 2018). Given that divergence time between Denisovans and MHs equals that between Neanderthals and MHs, it is likely that the fraction of Denisovan traits that we can predict using HPO phenotypes is high and similar to that in Neanderthals. The approach we presented relies on two basic hypotheses: (1) pronounced methylation changes (i.e., statistically significant unidirectional promoter methylation changes of >50% that extend across at least 50 CpG positions) are more likely to be associated with phenotypic effects than subtle changes, and (2) the direction of a phenotype driven by downregulation of a gene is expected to be similar to the direction of a phenotype driven by loss-of-function mutations. We demonstrated here that using these assumptions, we are able to reconstruct dozens of the traits that differ between human groups with over 80% accuracy. It might be surprising at first glance that the analysis of DNA methylation alone is sufficient to reach such accuracy. However, it was shown that there is high correlation and interplay between DNA methylation and other regulatory layers, such as transcription-factor binding and histone modifications (Banovich et al., 2014). Therefore, although this analysis was based on a single regulatory layer, the information that is extracted from it might reflect a sizeable portion of the regulatory landscape. Furthermore, as we did not reconstruct the extent of each phenotypic change, but rather only its direction of change, it is likely that the other regulatory layers, as well as protein changes and non-promoter methylation changes, further contribute quantitatively to the observed phenotypes. We conclude that unidirectional promoter methylation changes can be used to identify phenotypic divergence between closely related organisms. Even though we validated this approach on Neanderthals and chimpanzees, as well as on the Denisovan jawbone, its ultimate test would be to match the reconstructed profile to a more complete collection of Denisovan samples, once they are discovered. Cell, VOLUME 179, ISSUE 1, P180-192.E10.
|
|